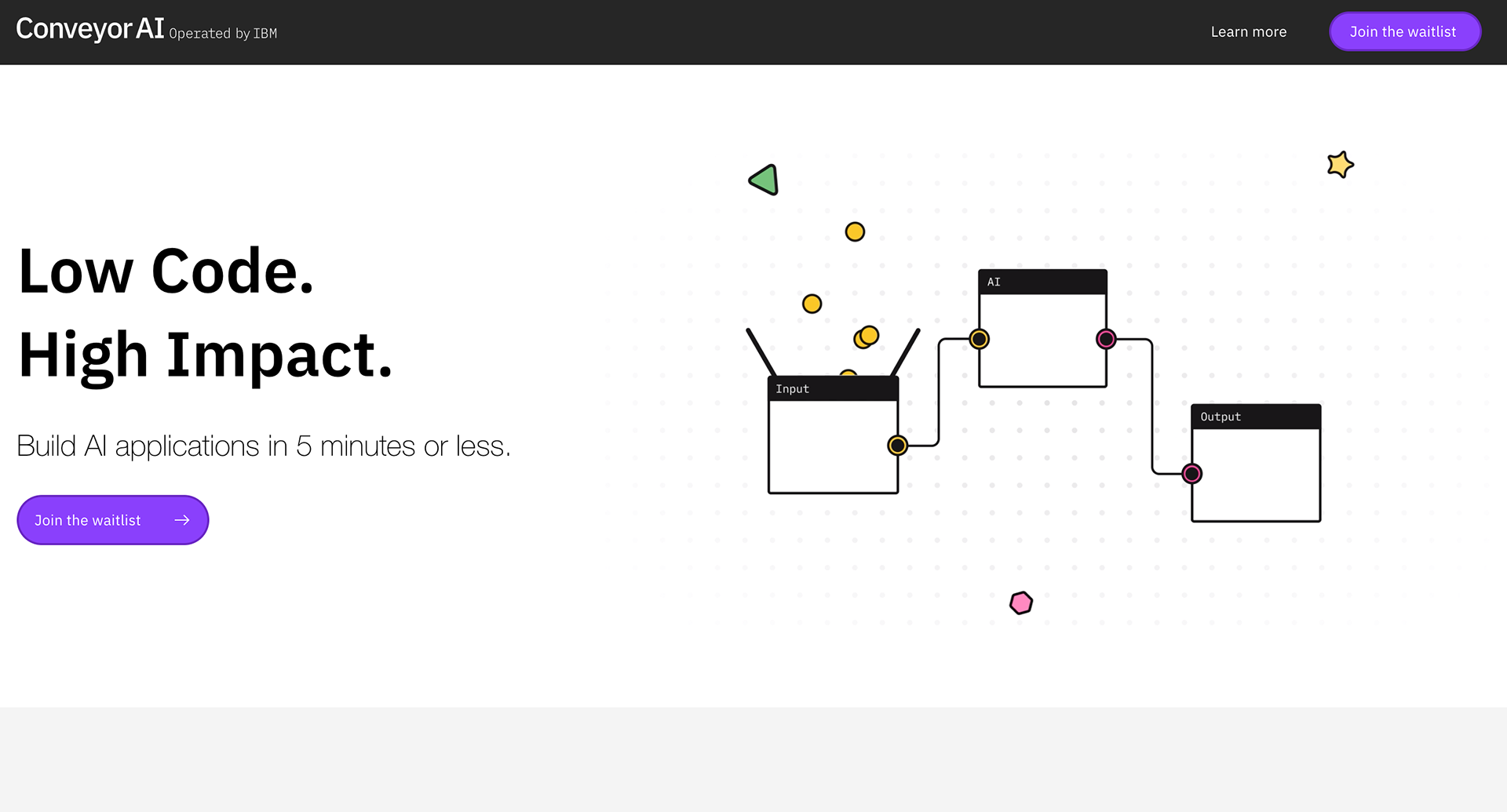
Background
At IBM, most designers get to go through an intense 6 week design thinking course, that challenges them to take on IBM's enterprise design thinking toolkit and apply it to real projects being worked on in the company.ย
This case study covers a project I worked on where myself and a team of 4 other designers helped Conveyor AI flesh out their product offeringย
๐
Duration: 4 weeks
๐ Tools: Figma, userzoom, mural, Webex
๐ Practices: Qualitative research (user testing / interviewing) , quantitative research (surveys), journey mapping, product design
Process
To kickoff the project, our team met with the Conveyor AI team, including their design team. We had a chance to hear from key stakeholders and understand their concerns, questions, and what they were hopeful for.ย
Additionally we learned that 2 other design teams had done a similar project to ours and we were building off of their work. It was up to us to synthesize all their work to date and understand what would be the right direction to move forward.
A key learning we gleaned from our discover sessions was that the team was concerned about the product once a user signs in.ย
How could they easily learn how to use the tool?ย
What happens if they get stuck?
Do they want to see AI templates?
What else do they want to see?
Getting Started
Making sense of past data ๐
Once we met with the stakeholders and understood their concerns, it was time to review the past design work done to date. Luckily for us, the previous teams did a good job of expanding upon the initial Conveyor AI prototype and also defined a working user persona based on internal stakeholder interviews as well as interviews with other AI developers at IBM.
Our working persona: Maria the developer ๐ฉโ๐ป
The previous design team identified "Maria" as the main persona for this tool. Maria is an intermediate developer working on a team trying to support other non development teams at their organization such as customer service teams trying to automate processes with AI. Unfortunately, Mariaย doesn't have the time needed to teach herself how to create AI models and struggles managing their work priorities to help her team out
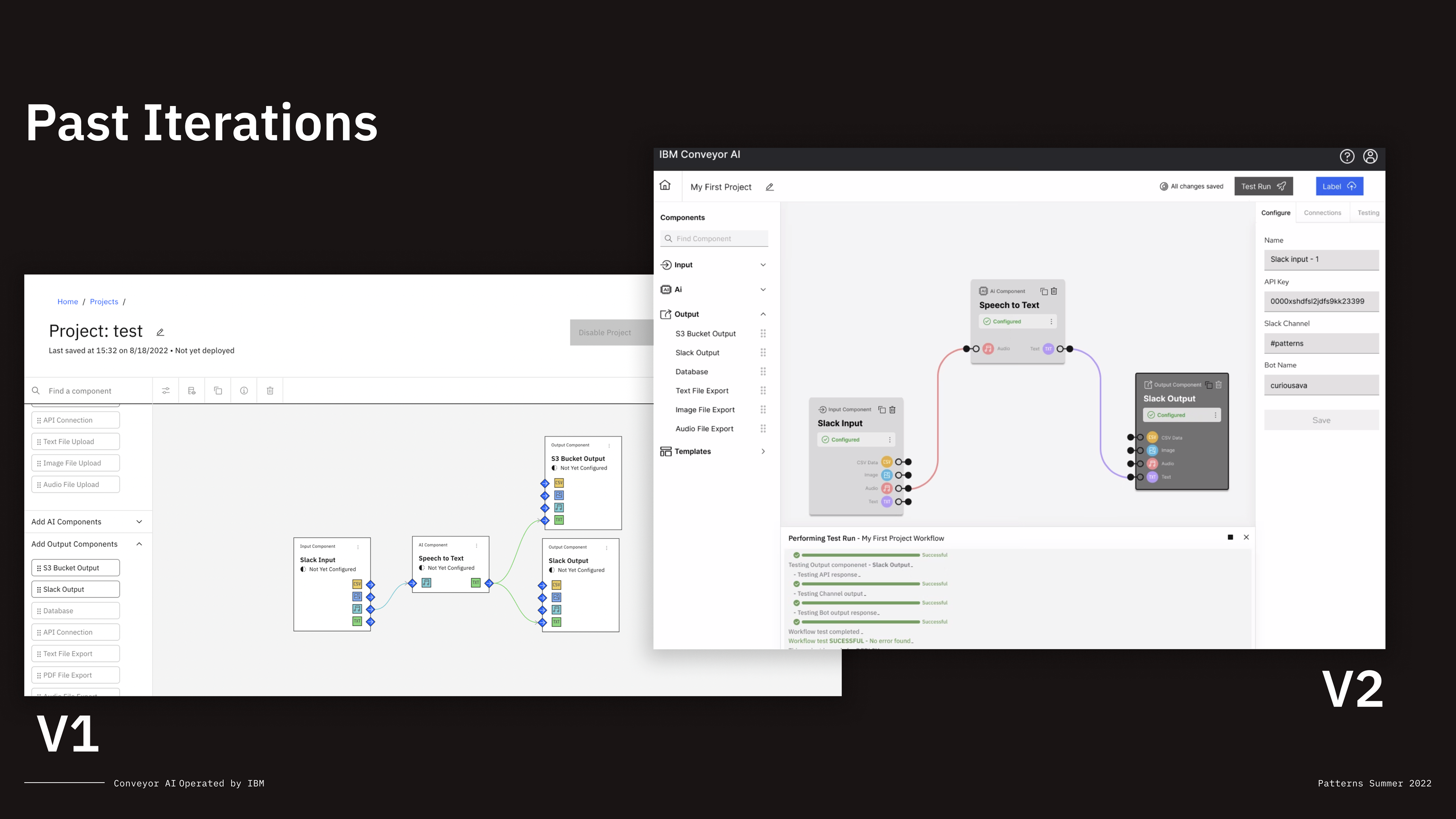
๐ Shown above are previous versions of the AI editor
Changes from v1 to v2 include:
- better panel structure for visibility to see AI inputs, outputs, and models
- the ability to view configurations without sub navigation
- better visual cues for file/project management
๐ To help us understand the existing oppurtunities to improve V2, we decided to do a journey mapping exercise as a team to figure out the steps required to sign in, get started with the tool, create a simple AI flow, test it, and deploy it
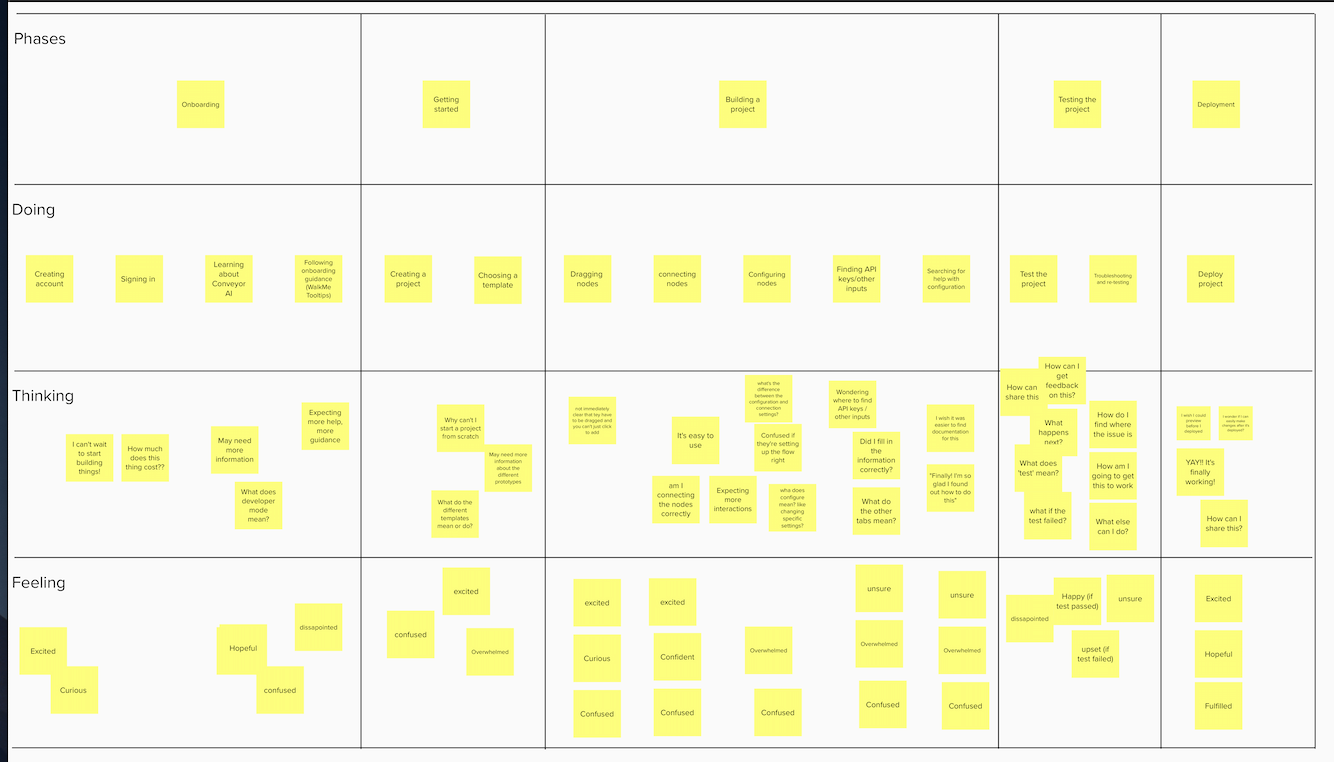
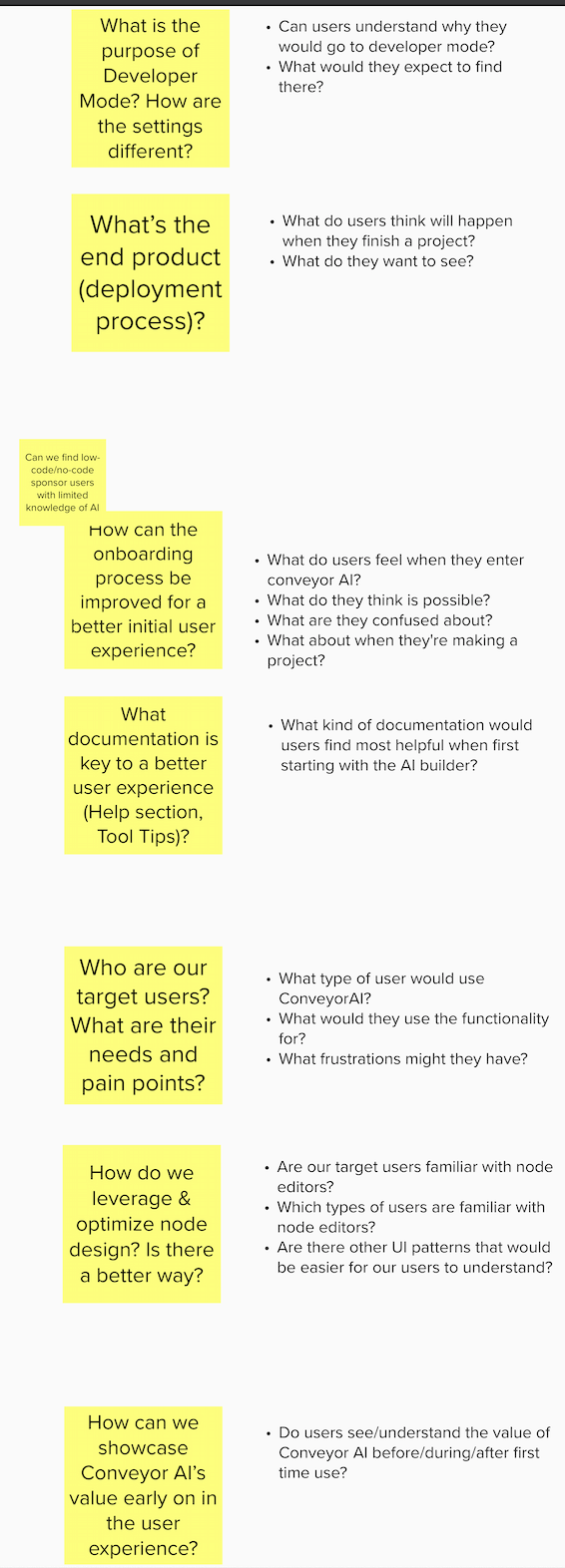
We hypothesized that while there is a lot of initial excitement to use the tool, there are a lot of times where the tool does nothing to guide the user and empower them to know exactly how it works. The user is kind of left alone to figure things out. We felt like this was a great thing to focus on in our 4 week sprint to validate and solve for.
Research Overview
After identifying our opportunity to improve, we agreed as a group on our core objectives and presented them back to the Conveyor AI team
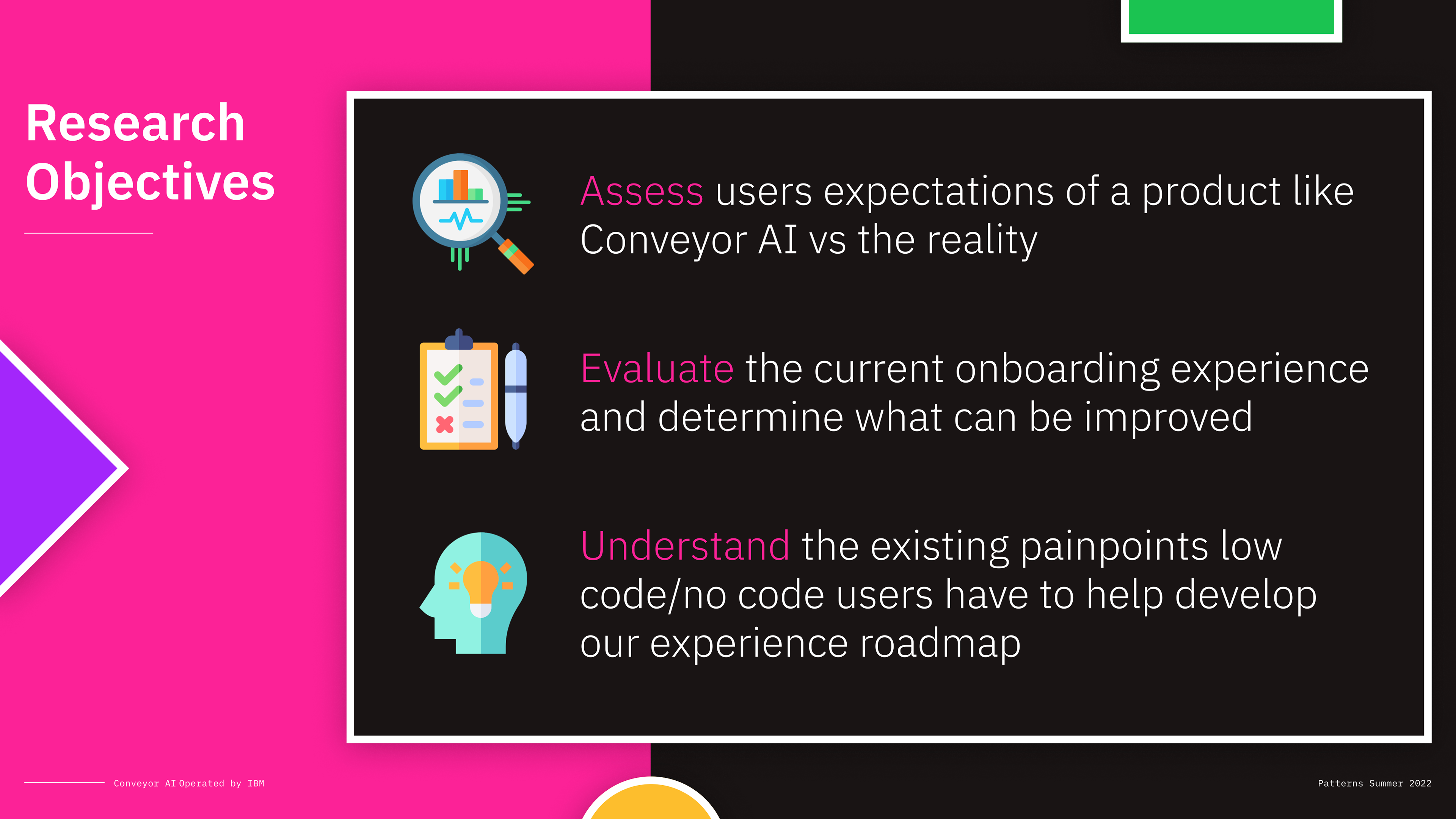
For context, we presented the team with our full plan in the form of a research plan document, outlining our hypotheses, desired approach, and other research materials ๐
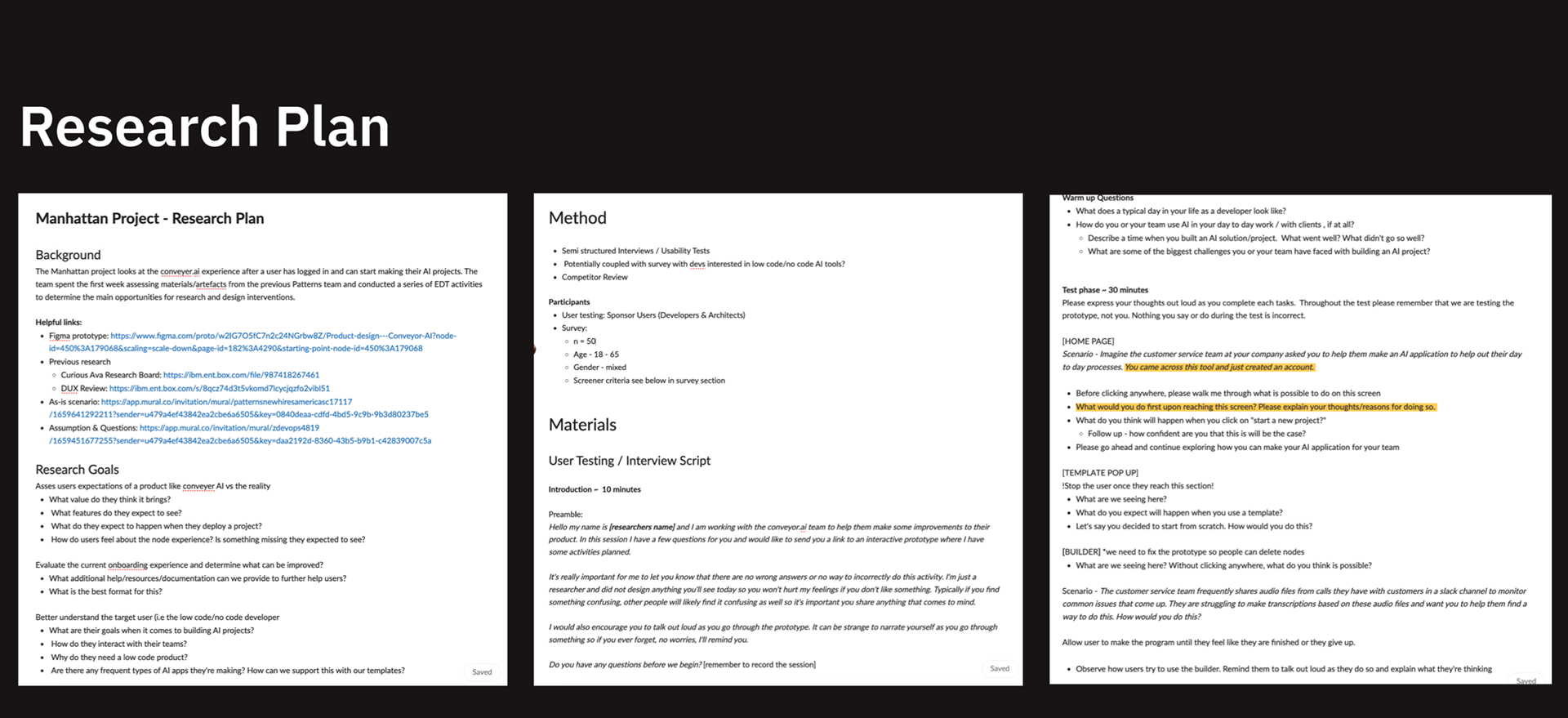
Because we had a lot of attitudinal information we needed to verify + we needed evaluateย the latest version of the experience, we decided to do semi structured interviews + usability testing via our prototype in Figma.
This way, we could understand their sentiments/expectations towards a tool like conveyor AI, understand their use cases, and also uncover other usability issues with the prototype itself, such as how they get started or how they would get help.
To help build our understanding of Maria, we also decided to run a survey with developers interested in using a low code/no code AI builder in their current workplace. We asked about their needs, competitors they have used, and also their requirements for adoption.ย
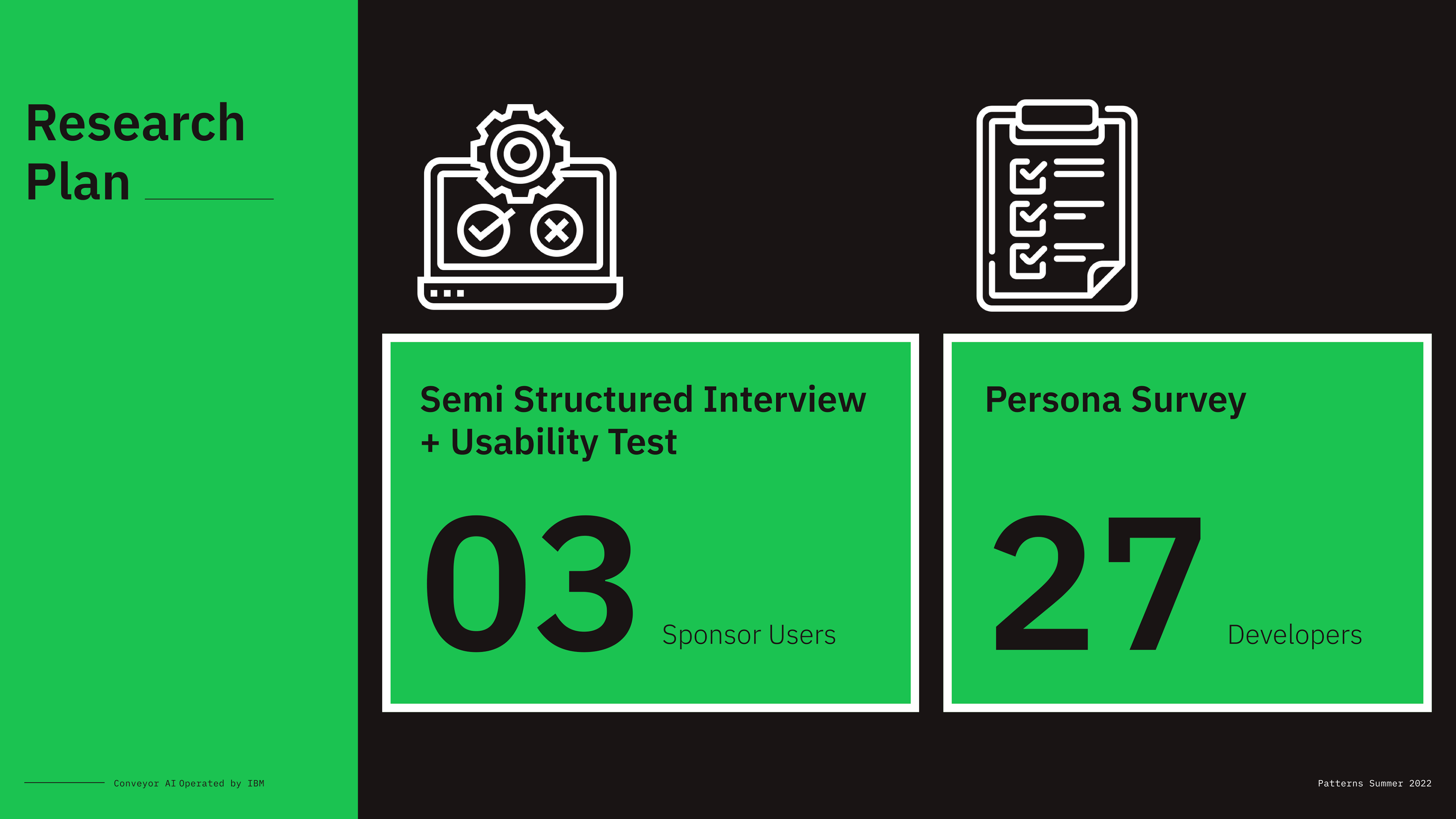
Data Synthesis ๐
After conducting our research, we met again as a team and conducted affinity mapping to map out the main things said in each interview and then group them based on themes. After that, we could get an idea for frequency in themes and that way we prioritized what are the biggest things we should focus on.
Additionally, while we validated our hypothesis in that users require more materials to get started faster, we also found several other equally important features that users would like to see in a product like Conveyor AI
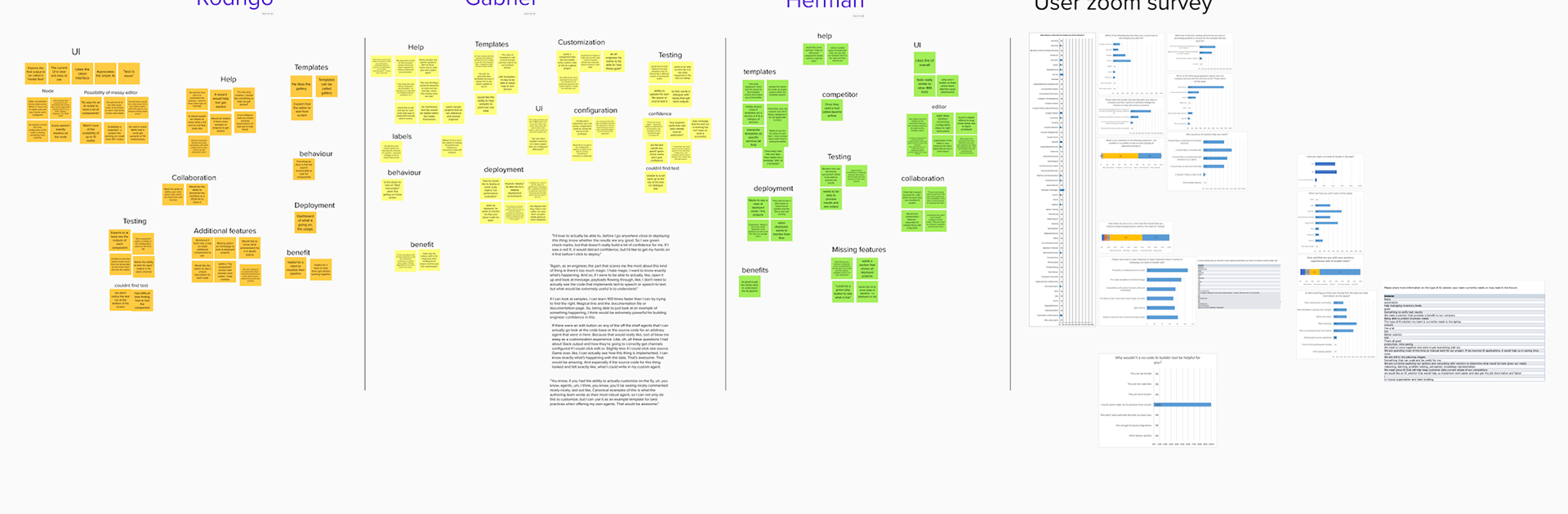
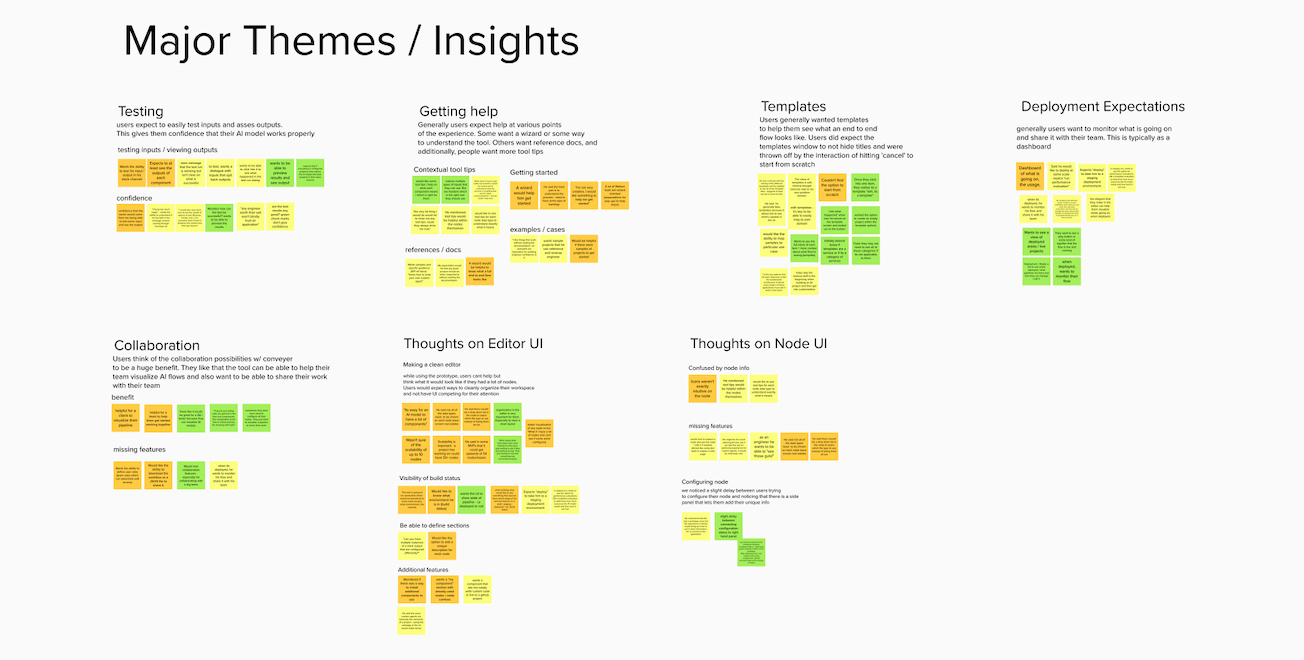
Insights
Updating our persona ๐ฉโ๐ป๐
Thanks to our survey, we had some more data to further iterate on our persona. We learned most people interesting in trying/buying a low code AI builder are actually more senior and not intermediate developers. This is because they have more say on vendors being used by their team. Additionally we were able to flesh out Maria's goals and frustrations.
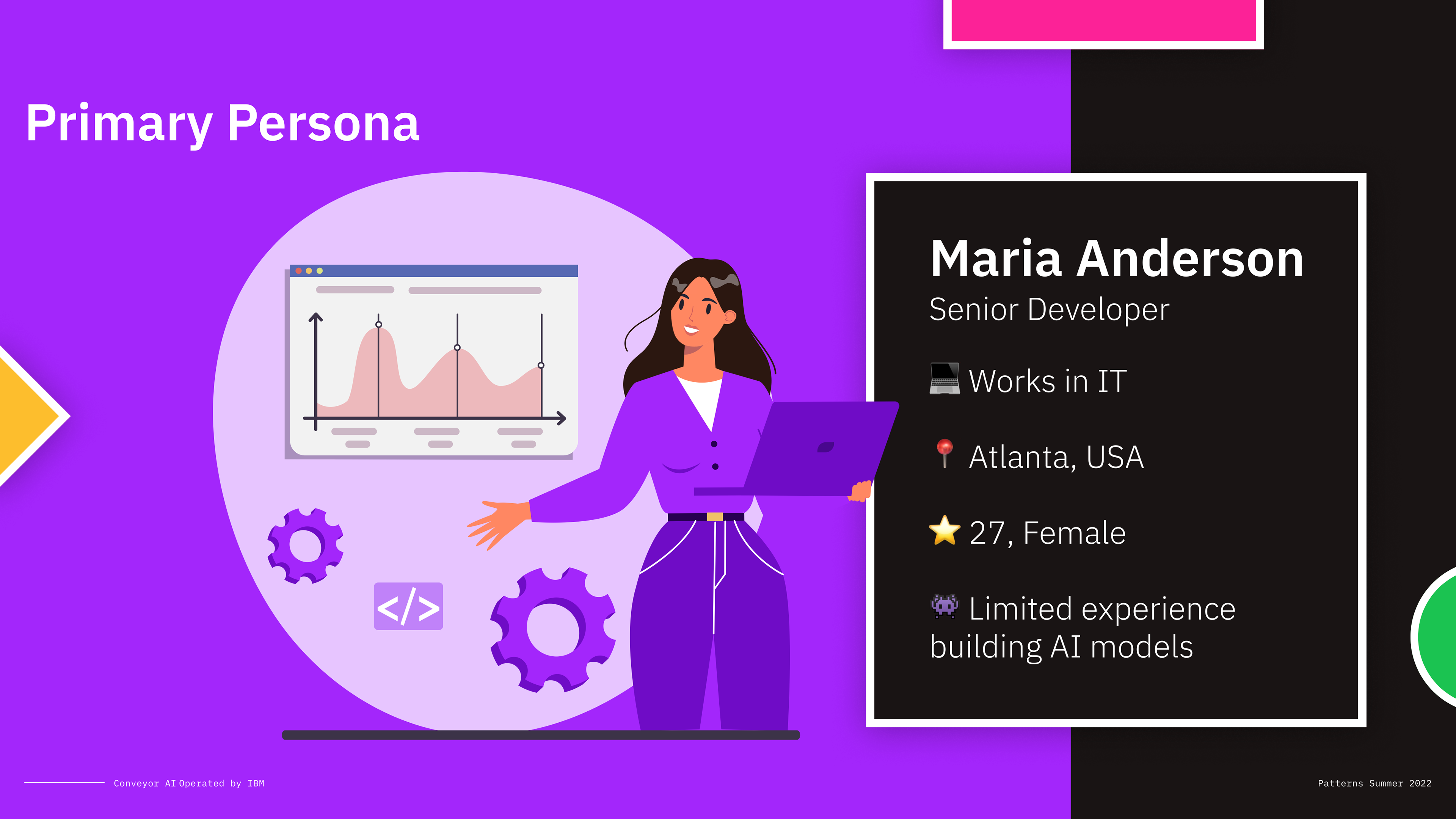
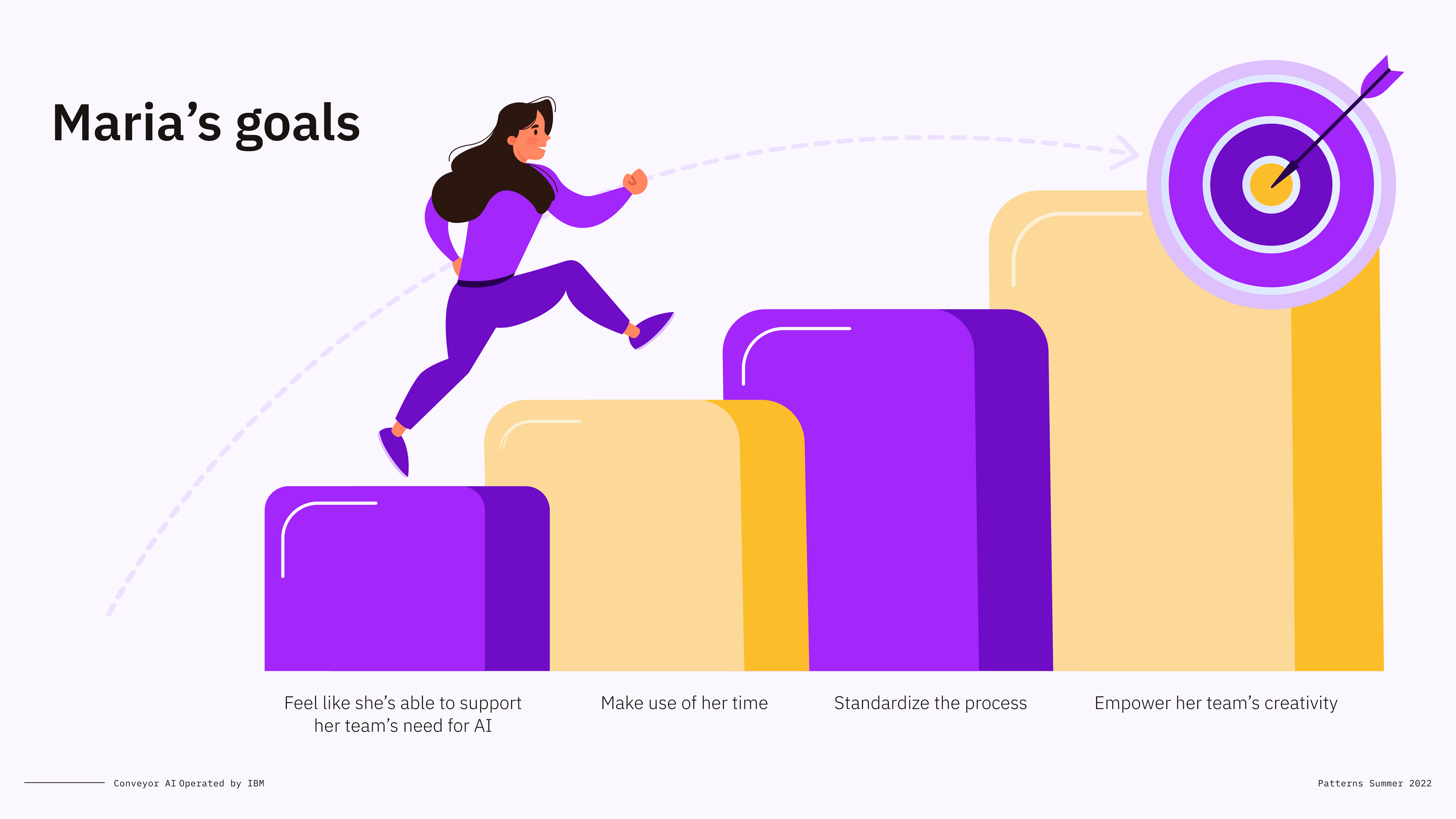
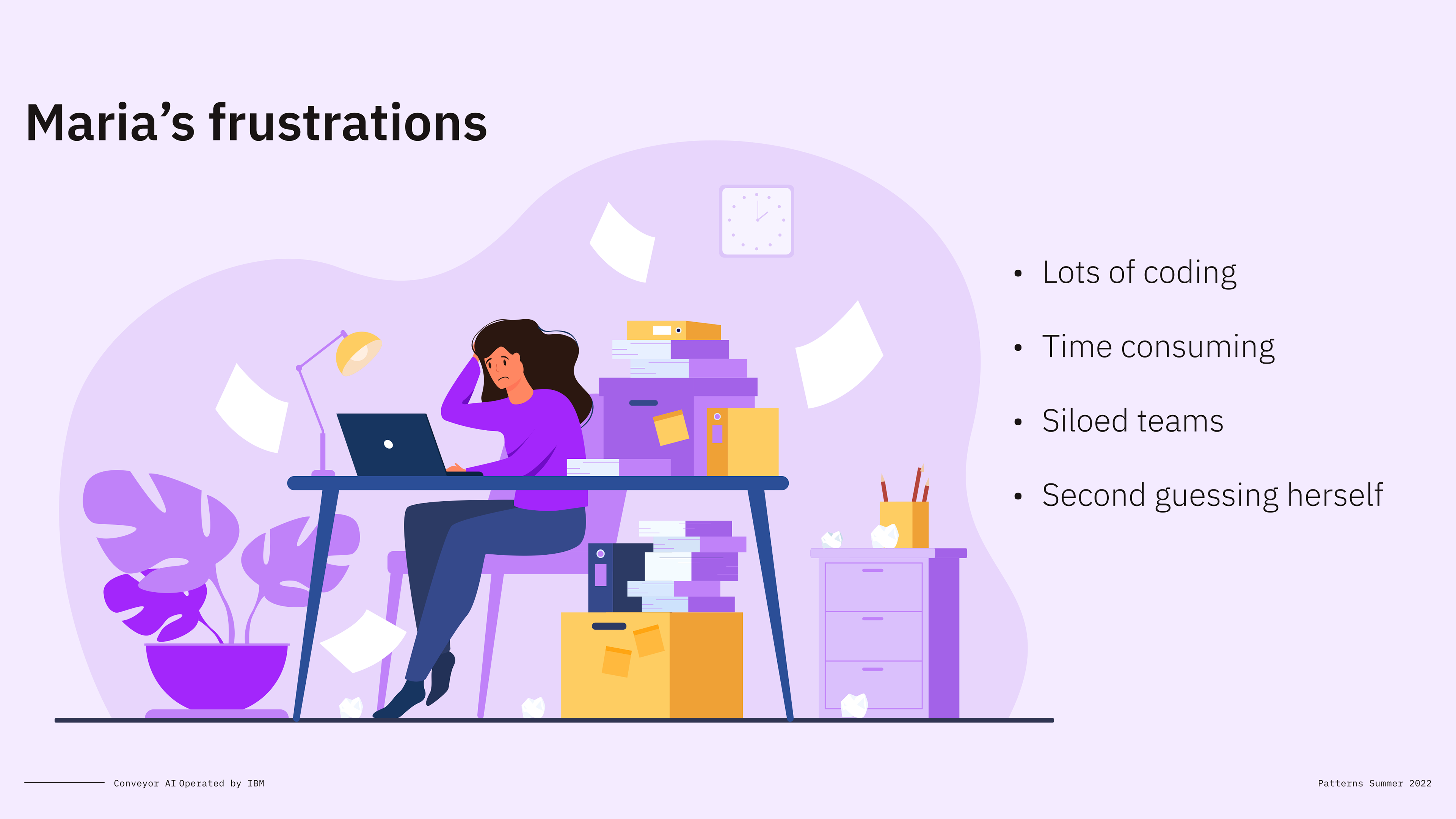
How users expect to get help ๐โโ๏ธ
Since we agreed as a team to focus our scope on onboarding, we decided to focus our delivery on those findings, and highlight our other findings as future items to focus on.
When it comes to creating confidence for using the tool, we learned it was't just 1 thing that could be included to do this. There were actually "modes" we needed to account for
1. initial learning -> we need to supply resources for tours, FAQs and active learning where users seek out info
2. Exploring -> a big part of a building tool is free exploration. We need to support this via having information that can be contextually placed in the form of tool tips
3. Understanding -> Developers don't always want to read documentation. They like to reverse engineer things. By providing templates and case studies, we allow for their own free learning/understanding
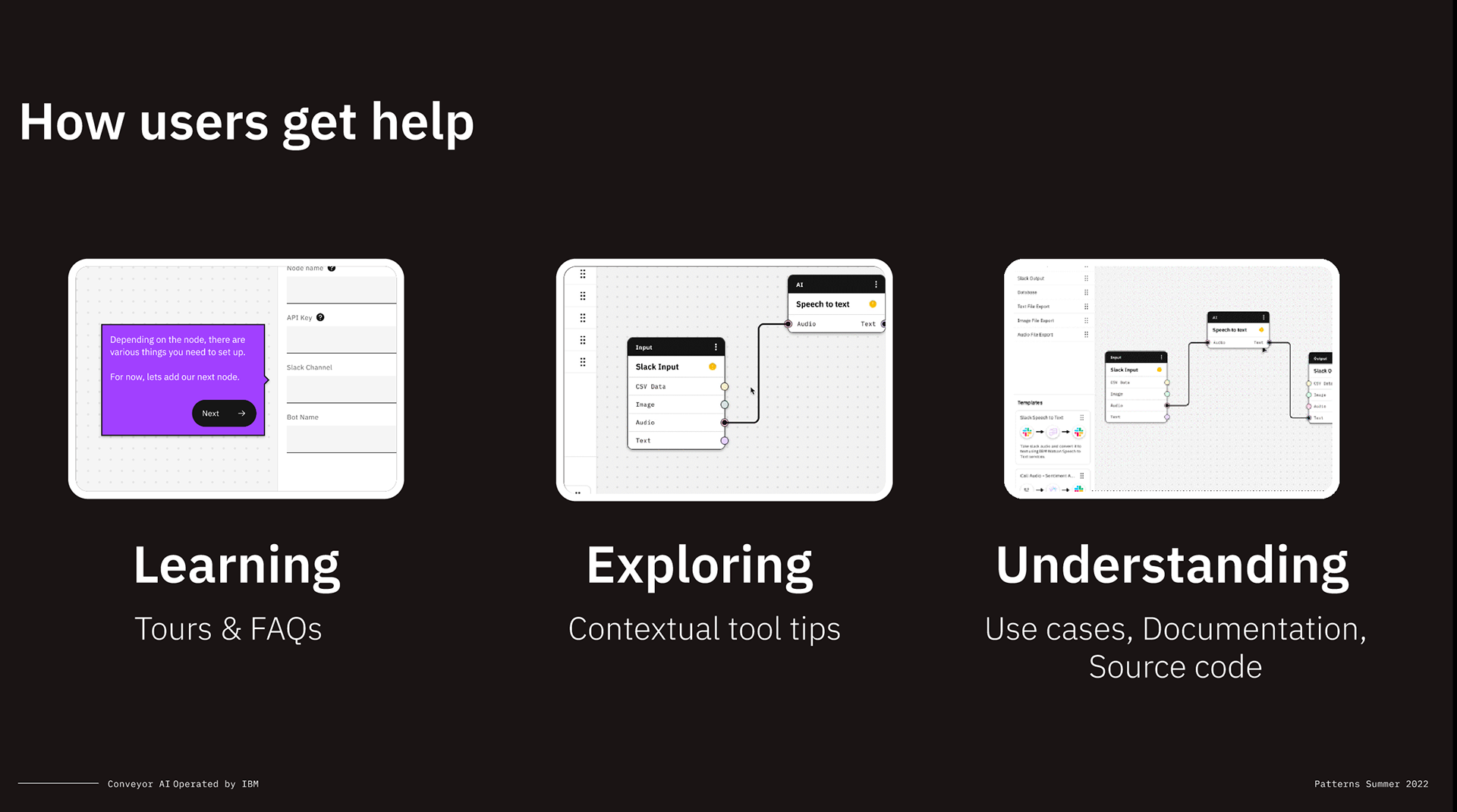
Future considerations ๐
Additionally we learned that the more we support visualization of actions/data, provide oppurtunities for customization, and encourage collaboration features, the more we create unique value propositions users want to see in a tool like this.
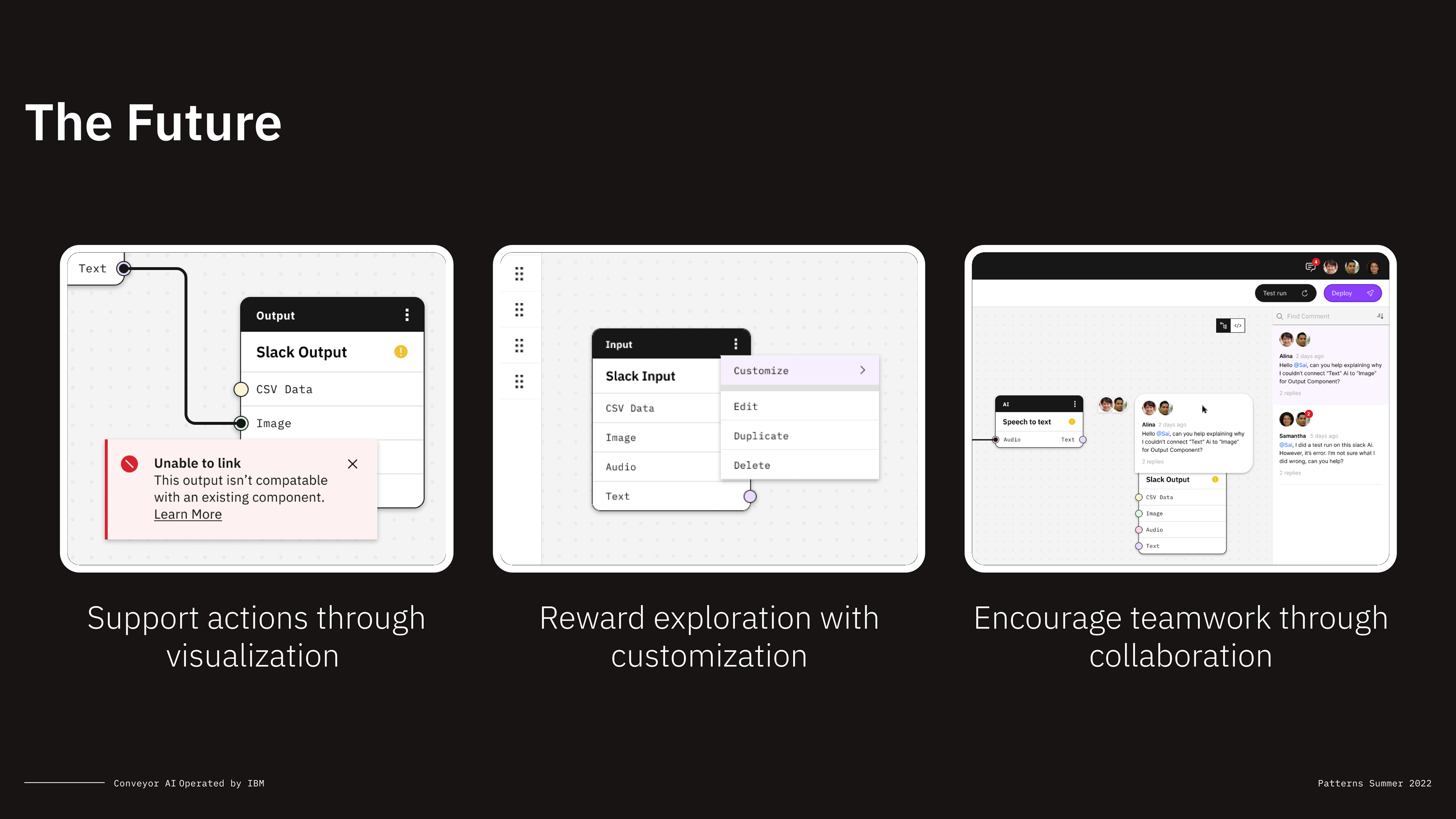
Output based on reseasrch
Refining our mission ๐ถ
We delivered the following user goal to the Conveyor AI team ๐
Maria should be able to become an expert at making applications with Conveyor AI within her first day of using it
Additionally:
- Maria should be able to make her first AI application in under 30 minutes
- Maria can find support for building her AI application in under 5 minutes
Methods of providing onboarding support to users ๐
ย One of the first things we did was introduce a guided onboarding tour for first time users. Our testing showed us that developers are mixed when it comes to directly wanting to get help. Some prefer guides while others prefer to do things themselves so we made sure oppurtunities to skip the tour were clear ๐
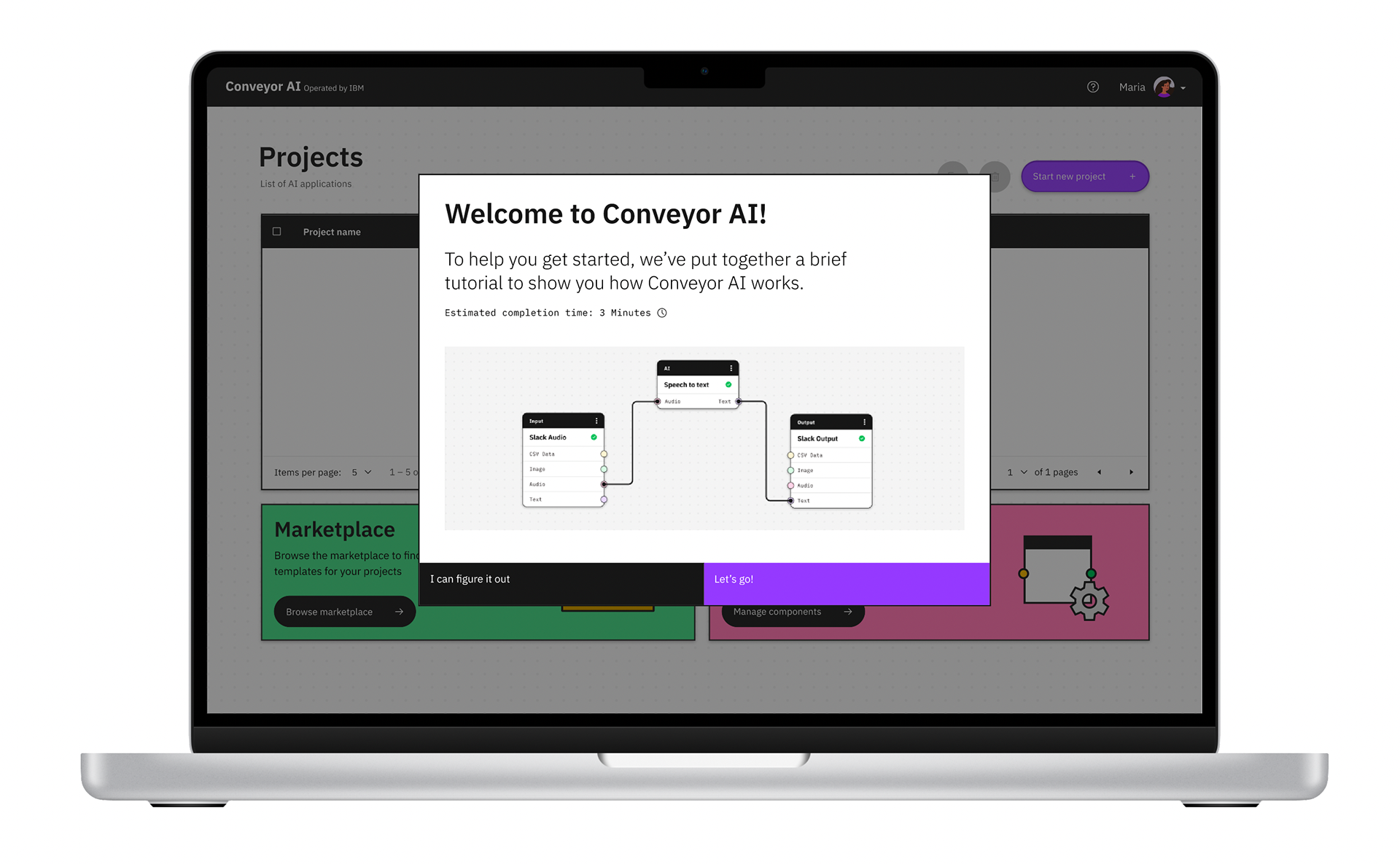
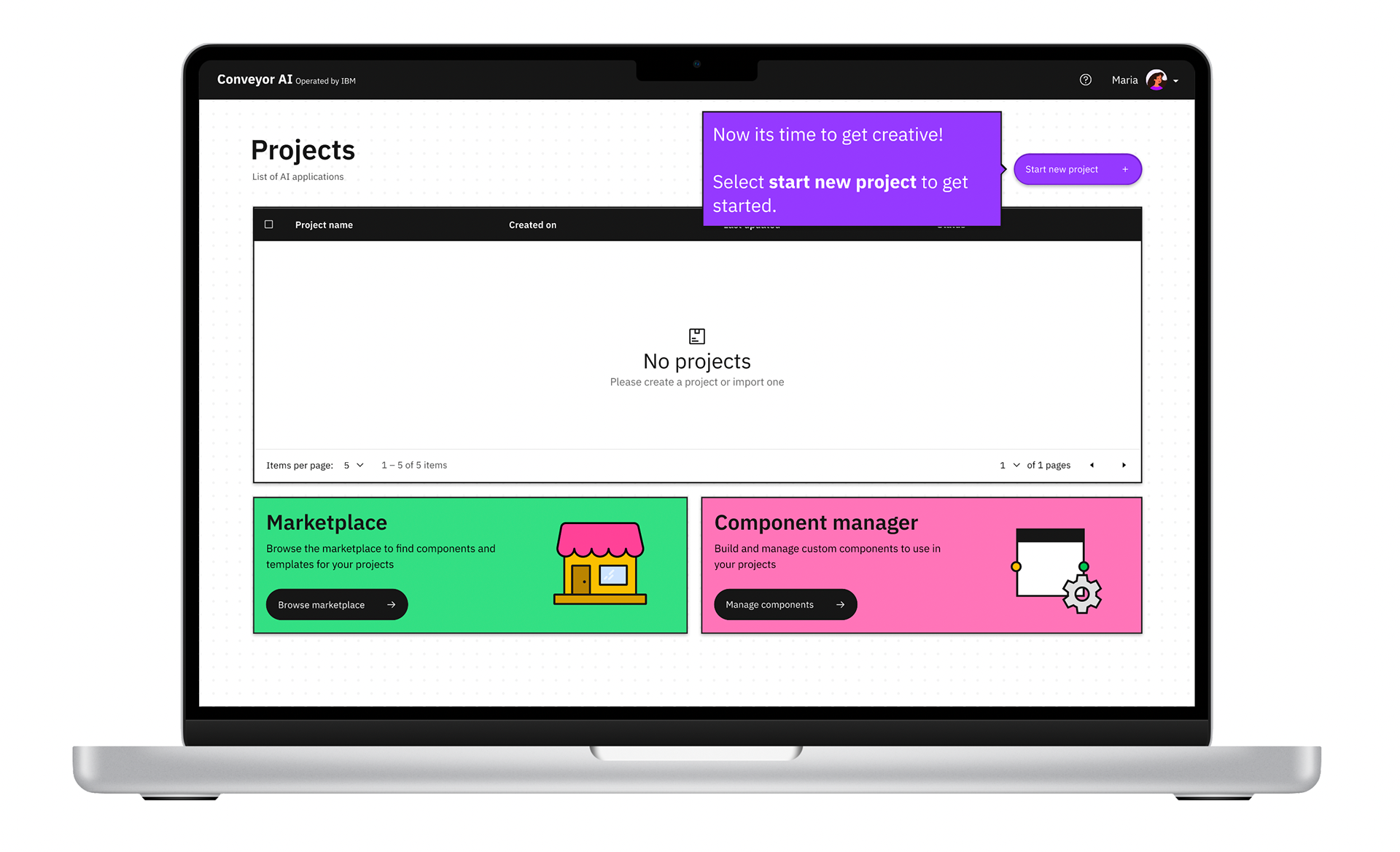
While the tour guided users to build and deploy their first AI model, we also introduced a variety of contextual tool tips throughout the builder. This supports free exploration and avoids giving the user too much information at once.
Tool tips cover things like statuses, inputs, requirements, acronyms etc. ๐. In some cases, tool tips take you to documentation for supported tools and APIs we support.
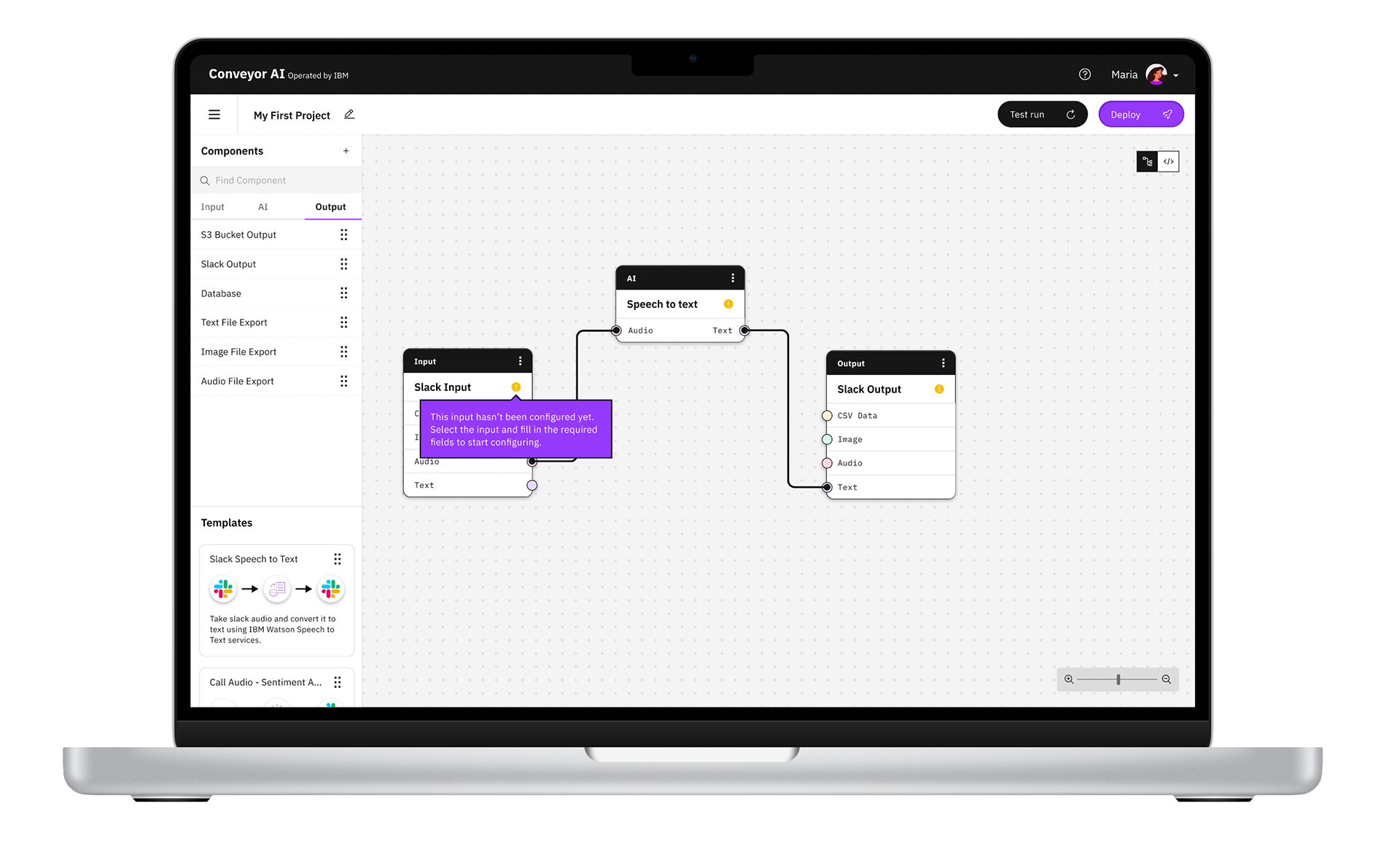
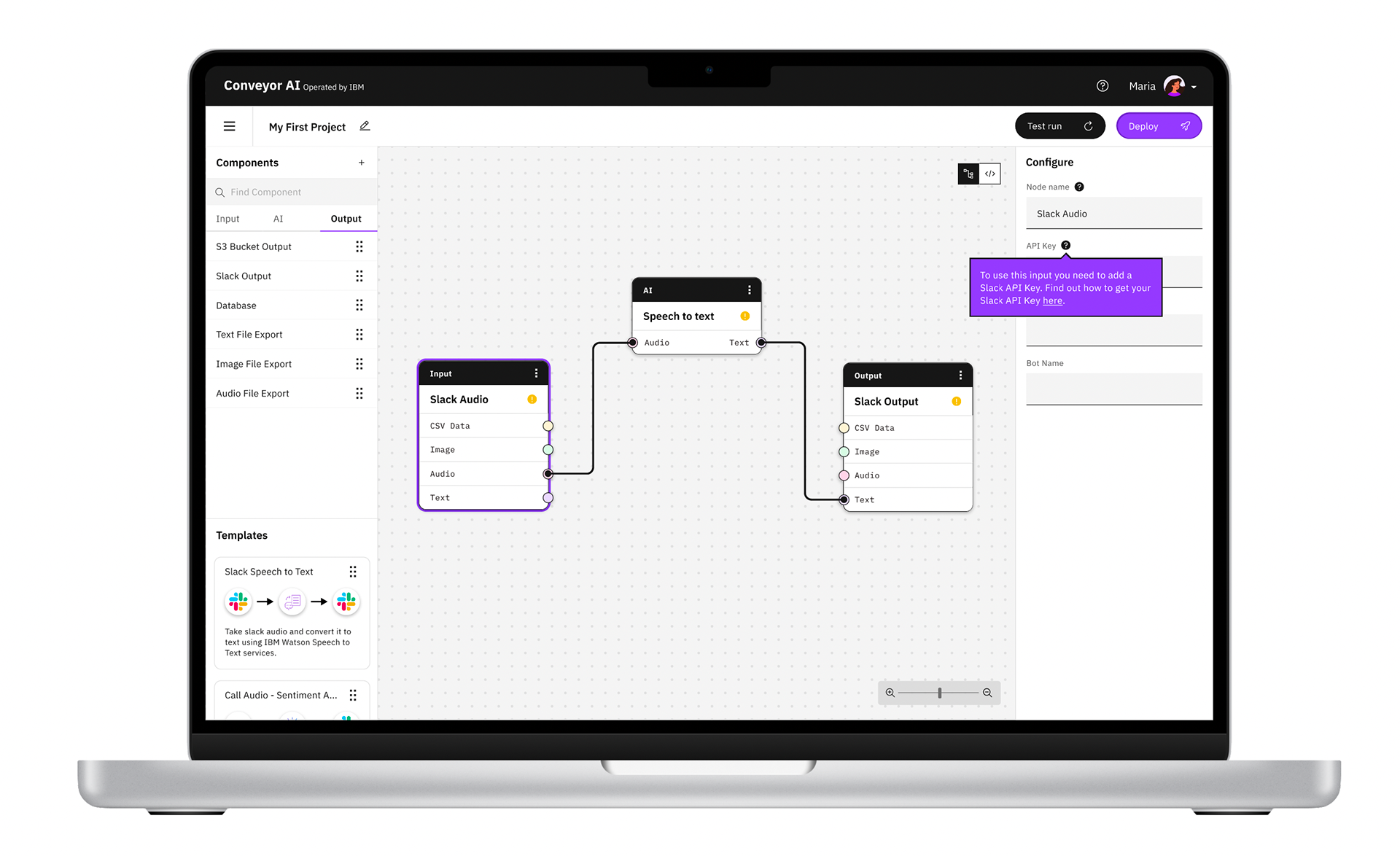
We also made templates much easier to find and use. In the previous iteration, templates were hidden in a menu. In this iteration, we brought it up to the surface level, and put it on the bottom of the land hand panel.ย
Furthermore, we made templates dynamic. They map to the type of input selected. This further boosts contextual learning, as the builder adopts to fit the users interests. ๐
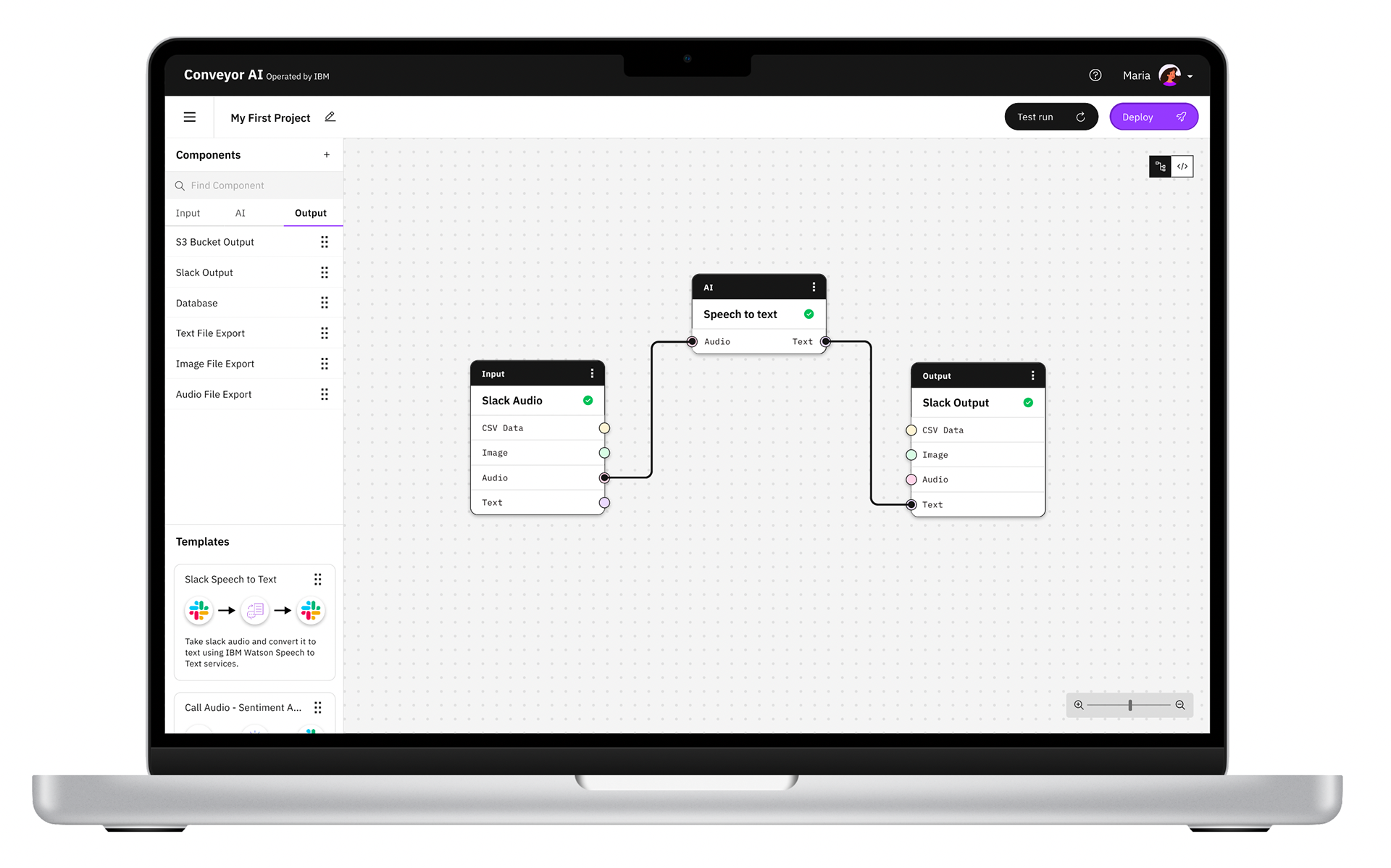
Lastly we included a help section that sits on the top right of the builder. This takes the user to an FAQ page and a way to contact support.
Next Steps
Conveyor.ai is seeking to launch soon and is using our designs in its first launch. Furthermore, our design direction is what is now featured on their public site.ย
You can view it here